In a recent study published in the Scientific Reports Journal, researchers used a machine learning (ML)-based approach to predict adulthood obesity by assessing risk factors and tracking body mass index (BMI) values in the initial 1,000 days (between two and four years of age) of life.
Study: Predicting body mass index in early childhood using data from the first 1000 days. Image Credit: NicoElNino/Shutterstock.com
Background
Obesity prevalence has considerably increased across the globe among adults and children. Early adiposity among pediatric individuals predicts adult obesity, cardiometabolic risks, and pediatric morbidities.
After establishment, obesity is difficult to treat and likely to persist. Therefore, research prioritizes obesity prevention, and detecting individuals at a heightened risk of adiposity during adulthood could improve prevention efforts.
Modifiable risk factors include higher BMI values for mothers before pregnancy, weight gain during pregnancy, low socioeconomic status, high neonatal weight, and neighborhood-level variables (such as crime and food accessibility). However, data on the combined risk estimation potential of the variables are limited.
Existing efforts that estimate pediatric obesity, including factors that enhance obesity risks in the antenatal and initial neonatal periods, are few, despite studies reporting that two to four years of age offer higher developmental pliability and health behavior-influencing opportunities.
About the study
In the present study, researchers used ML algorithms to identify children at an increased risk of obesity, which could inform obesity prevention policymaking and strategy development. They also devised a dynamic, predictive BMI tracker to be used during childhood to identify the risk of adulthood obesity.
The team used the least absolute shrinkage and selection operator (LASSO) regression for retaining features with the highest coefficients and relevance to pediatric obesity other than height, weight, and body mass index.
They developed estimation models using support vector regression (SVR) with fivefold cross-validation to estimate BMI at 30 to 36 months (4,204 individuals), 36 to 42 months (4,130 individuals), and 42 to 48 months (2,880 individuals). The team excluded individuals without ≥1.0 clinical encounters in all periods.
The steps involved in model development were obtaining and integrating raw data, pre-processing data, feature engineering, training, and tracker validation. The tracker was trained using 80.0% of individuals’ data (training dataset) from all periods.
Electronic health records (EHRs), birth certificates, and geocoded data were retrieved from the Obesity Prediction in Early Life (OPEL) registry from 2004 to 2019. The study outcome was BMI based on participant age and gender, according to the Centers for Disease Control and Prevention (CDC) recommendations.
Results
The OPEL registry comprised 149,625 visits for 19,724 individuals aged 0.0 months to 48.0 months, of which 10,348 individuals were analyzed, among whom 4,204, 4,130, and 2,880 were aged 30.0 to 36.0 months, 36.0 to 42.0 months, and 42.0 to 48.0 months.
Eliminating erroneous records, imputing missing values, and scaling exposure variables, 50 variables were selected. After LASSO regression, data augmentation, and univariate tests, 19 variables were analyzed.
The model comprised the following variables: mean height, BMI, and weight at 0.0 to 8.0 months, 8.0 to 16 months, and 16 to 24 months; time differences between the final encounter during the periods and that before two years; mean age, weight, height, BMI, and weight and height percentiles at two years; estimation time differences between the final visit before two years and target visit during either of the periods.
Testing the tracker using the validation dataset (20.0% of patients) showed an accurate estimation of childhood BMI (mean error of 1.0 at 30.0 to 36.0 months, 36.0 to 42.0 months, and 42.0 to 48.0 months).
Most variables in the model showed significant correlations with pediatric BMI across all estimation ranges. The findings indicated that the tracker could support clinicians’ and population-level efforts to prevent obesity during the initial days of life.
Modifiable factors related to higher childhood BMI were detected in the prenatal and initial infancy stages, including maternal risk factors during pregnancy, C-section delivery, greater infant weight at birth, and whether the infant wakes up at night and requires assistance to fall asleep.
Factors such as the proportion of individuals residing in food deserts and Hispanic ethnicity protected against elevated BMI.
Conclusions
Overall, the study findings showed that pediatric BMI trajectories could be assessed using ML and modifiable risk factors during early childhood, supporting efforts to intervene before the onset of unhealthy adiposity to reduce the health burden of obesity.
Maternal health, the quality of a child’s sleep, and socioeconomic factors could influence children’s weight trajectories during later childhood.
Unlike existing models that estimate BMI using weight cut-offs at particular time points, the body mass index tracker could predict BMI in three future six-month intervals (i.e., 30.0 to 36.0 months, 36.0 to 42.0 months, and 42.0 to 48.0 months).
The findings could enable pediatric providers to observe changes in BMI over extended periods.
-
Cheng, E., Cengiz, A. and Miled, Z. (2023) "Predicting body mass index in early childhood using data from the first 1000 days", Scientific Reports, 13(1). doi: 10.1038/s41598-023-35935-6. https://www.nature.com/articles/s41598-023-35935-6
Posted in: Child Health News | Medical Science News | Medical Research News | Medical Condition News | Healthcare News
Tags: Body Mass Index, Cardiometabolic, Children, C-section, Food, Machine Learning, Maternal Health, Obesity, Pregnancy, Prenatal, Research, Sleep
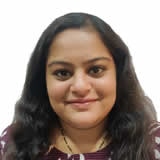
Written by
Pooja Toshniwal Paharia
Dr. based clinical-radiological diagnosis and management of oral lesions and conditions and associated maxillofacial disorders.
Source: Read Full Article