In a recent Nature Medicine journal study, researchers develop an artificial intelligence (AI)-based model to detect Parkinson’s disease (PD) and track its progression from nocturnal breathing signals.
Study: Artificial intelligence-enabled detection and assessment of Parkinson’s disease using nocturnal breathing signals. Image Credit: Natali _ Mis / Shutterstock.com
Background
Since PD is the fastest-growing neurological disease worldwide, there is an urgent need for novel diagnostic biomarkers that can detect the disease at an early stage. Currently, there are no drugs capable of reversing or ceasing PD progression. Furthermore, PD is typically diagnosed based on changes in motor functions, such as tremors and rigidity.
The assessment of PD progression primarily relies on patient self-reporting; however, clinicians also use the Movement Disorder Society Unified Parkinson’s Disease Rating Scale (MDS-UPDRS) for qualitative PD assessment.
Some existing PD biomarkers, including cerebrospinal fluid, blood biochemical, and neuroimaging, have shown promising results for their potential utility in the early diagnosis of this disease. However, these are expensive measures that require access to multispeciality hospitals, thus making them unsuitable for early diagnosis or PD progression tracking.
Renowned English surgeon James Parkinson first noted a connection between PD and breathing in 1817. Subsequent studies also reported degenerated brainstem areas that control breathing among PD patients, thus making breathing a promising risk assessment attribute for the clinical diagnosis of PD. Importantly, respiratory symptoms often manifest much before clinical motor symptoms of PD.
About the study
In the present study, researchers evaluated a novel AI-based study model on 7,671 individuals using data from several public datasets and hospitals in the United States.
The study model gathered data in two distinct ways. The first method required that the test subject wore a breathing belt on the chest or abdomen to track breathing signals overnight. The data acquired from this method was referred to as the breathing belt dataset.
Alternatively, the second contactless method collected breathing data using a radio sensor that transmitted a low-power radio signal in the individual’s bedroom and analyzed its reflections from the environment. This dataset was referred to as wireless data.
The model learned the auxiliary task of predicting each subject's quantitative electroencephalogram (qEEG) from nocturnal breathing. Notably, the researchers excluded subjects from testing who helped train the neural network.
The team performed k-fold cross-validation (k equals four) for PD detection and leave-one-out validation for severity prediction. Cross-institution prediction was determined by training and testing the model on data from different medical centers.
The AI model diagnosed PD from one night of nocturnal breathing, which was presented as the receiver operating characteristic (ROC) curve. Although the AI model detected PD with high accuracy, the researchers were interested in assessing whether its accuracy was improved by combining data from several nights in the same test subject. Wireless datasets were used to compute the model prediction score for all nights.
The test-retest reliability was determined by averaging the prediction across consecutive nights within a pre-specified timeframe. Lastly, the ability of the AI model to generate a PD severity score that correlated with the MDS-UPDRS was evaluated.
Study findings
The mean age of the PD patients was 69.1 years, with 27% of the study participants being women. The control group consisted of 6,914 subjects, 30% of whom were women with a mean age of 66.2 years.
Longitudinal data of several nights for each person for up to one year were collected. Taken together, the combined study data included 11,964 nights with over 120,000 hours of nocturnal breathing signals from 757 PD patients.
The breathing belt datasets lacked MDS-UPDRS and Hoehn and Yahr (H&Y) scores. Conversely, the wireless datasets had MDS-UPDRS and H&Y scores.
The study model attained an area under the ROC curve (AUC) of 0.889 with a sensitivity and specificity of 80.22% and 78.62%, respectively, all with a 95% confidence interval (CI) for nights measured using a breathing belt. For nights measured using a wireless dataset, the model reached an AUC of 0.906 with a sensitivity and specificity of 86.23% and 82.83%, respectively.
The PD prediction score could be any number between zero and one. Only when the PD score predicted by the AI model exceeds 0.5, a person is considered to have PD. Therefore, the researchers used the median PD score for each subject as the final diagnosis result.
Combining several nights for each subject increased the sensitivity and specificity of PD diagnosis to 100% for both PD and control subjects. The reliability also improved and attained 0.95 in just 12 nights upon using data from several nights from the same subject.
A strong correlation between the AI models' severity prediction and MDS-UPDRS was observed, thus indicating that the model captured PD disease severity well. Since there was a strong correlation between model prediction and three subparts of MDS-UPDRS, with R values of 0.84, 0.91, and 0.93, it was determined that the model captured non-motor and motor symptoms of PD.
Conclusions
The AI-based system described in the current study served as a promising diagnostic and progression digital biomarker for PD. Moreover, the model was remarkably objective, non-obtrusive, inexpensive, and had the potential to allow nocturnal breathing measurements to be obtained repeatedly at home.
About 40% of PD patients currently do not receive care from PD specialists, as these clinicians are often concentrated in medical centers in urban areas. For such cases, as well as those at high risk of developing PD, the latter of which includes those with leucine-rich repeat kinase 2 gene mutation, an AI system could be deployed at home for passive tracking. Furthermore, this type of system could provide regular feedback to the patient’s doctor who could follow up with the patient to confirm the results through telehealth or in-person visit.
Such advances in AI can support medicine by addressing unresolved challenges in neuroscience research and providing new clinical insights for diagnosing and tracking PD progression.
- Yang, Y., Yuan, Y., Zhang, G., et al. (2022). Artificial intelligence-enabled detection and assessment of Parkinson’s disease using nocturnal breathing signals. Nature Medicine. doi:10.1038/s41591-022-01932-x
Posted in: Device / Technology News | Medical Science News | Medical Research News | Medical Condition News
Tags: Artificial Intelligence, Biomarker, Blood, Breathing, Diagnostic, Doctor, Drugs, Gene, Kinase, Leucine, Medicine, Movement Disorder, Mutation, Neuroimaging, Neurological Disease, Neuroscience, Parkinson's Disease, Research, Respiratory
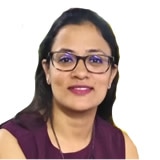
Written by
Neha Mathur
Neha is a digital marketing professional based in Gurugram, India. She has a Master’s degree from the University of Rajasthan with a specialization in Biotechnology in 2008. She has experience in pre-clinical research as part of her research project in The Department of Toxicology at the prestigious Central Drug Research Institute (CDRI), Lucknow, India. She also holds a certification in C++ programming.
Source: Read Full Article